Le laboratoire Georges Friedel vous invite à sa 1ère conférence-événement !Le 16 juin, le laboratoire…
Séminaire LGF – Johann Lüder (NSYSU, Taïwan) – 16 janvier 2025
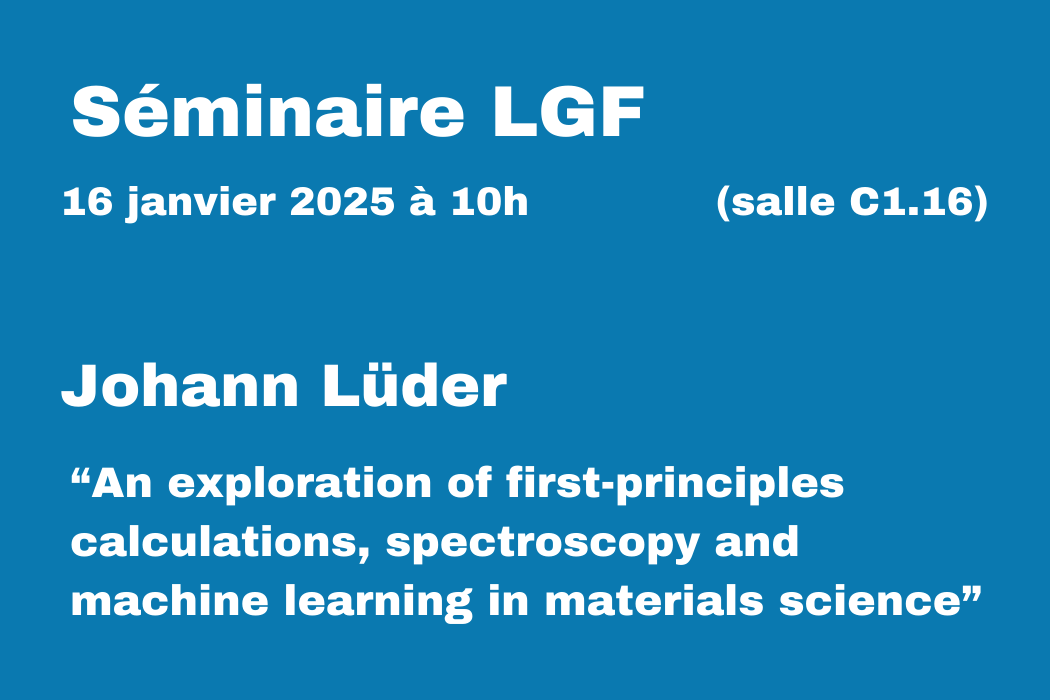
Intitulé
An exploration of first-principles calculations, spectroscopy and machine learning in materials science
Abstract
Materials research is at the frontier of technological innovations urgently needed to tackle many problems of modern societies, from an exponential increase of transmitted, stored, and processed data, to environmental pollution, sustainable resource management, clean energy generation, storage, and transfer. Many fundamental questions and possible solutions are today accessible and made possible through computational materials research contributing to, for instance, improved catalyst design for hydrogen economy, decarbonization and photocatalytic fuel generation. This talk will share our recent computational studies and developments in interfacing materials science, machine-learning techniques and first-principles calculations, and contributions to experimental studies. We will introduce our neural-networked-based development for X-ray adsorption spectroscopy at the L-edge and outline recent developments in Orbital-free Density Functional theory that apply a high-throughput database generation and a machine-learned kinetic energy functional. Furthermore, we introduce the cheminformatics concepts and possibilities of computational guided molecular design for clean energy devices including batteries and solar cells, and calculations of catalytic processes on metal and semiconductor surfaces.
Bio
Dr. Johann Lüder is an associate professor at the Department of Materials and Optoelectronic Science and an executive member of the Center for Theoretical and Computational Physics at National Sun Yat-Sen University (NSYSU) in Kaohsiung where he started to build his computational research group in 2018. In 2016, he joined the National University of Singapore for a two-year research fellowship stay at the Department of Mechanical Engineering after the completion of his PhD studies in Atomic, Molecular, and Condensed Matter Physics at Uppsala University in Sweden. He obtained his B.Sc. degree from Free University Berlin, Germany, and his M.Sc. degree from Uppsala University.
He develops machine learning approaches to advance computational material science methods, including theoretical X-ray spectroscopy simulation and first-principles methods such as Density Functional Theory (DFT) and Orbital-free DFT paving the wave for seamless first-principles calculation to explore phenomena spanning the atomic- and mesoscale. His computational lab applies these methods and combines them with high-throughput and data science techniques to derive a fundamental understanding and knowledge of materials’ properties on the atomic scale. The computational results can elucidate questions in sensor development, catalysis, clean energy conversion, and storage providing insights into the design of more durable and better-performing devices.